What Is Real-Time Data, and Why Does It Matter for Retail?
Learn how choosing real-time data over static data can solve data latency, improve retail data analytics, and inform better business decisions.

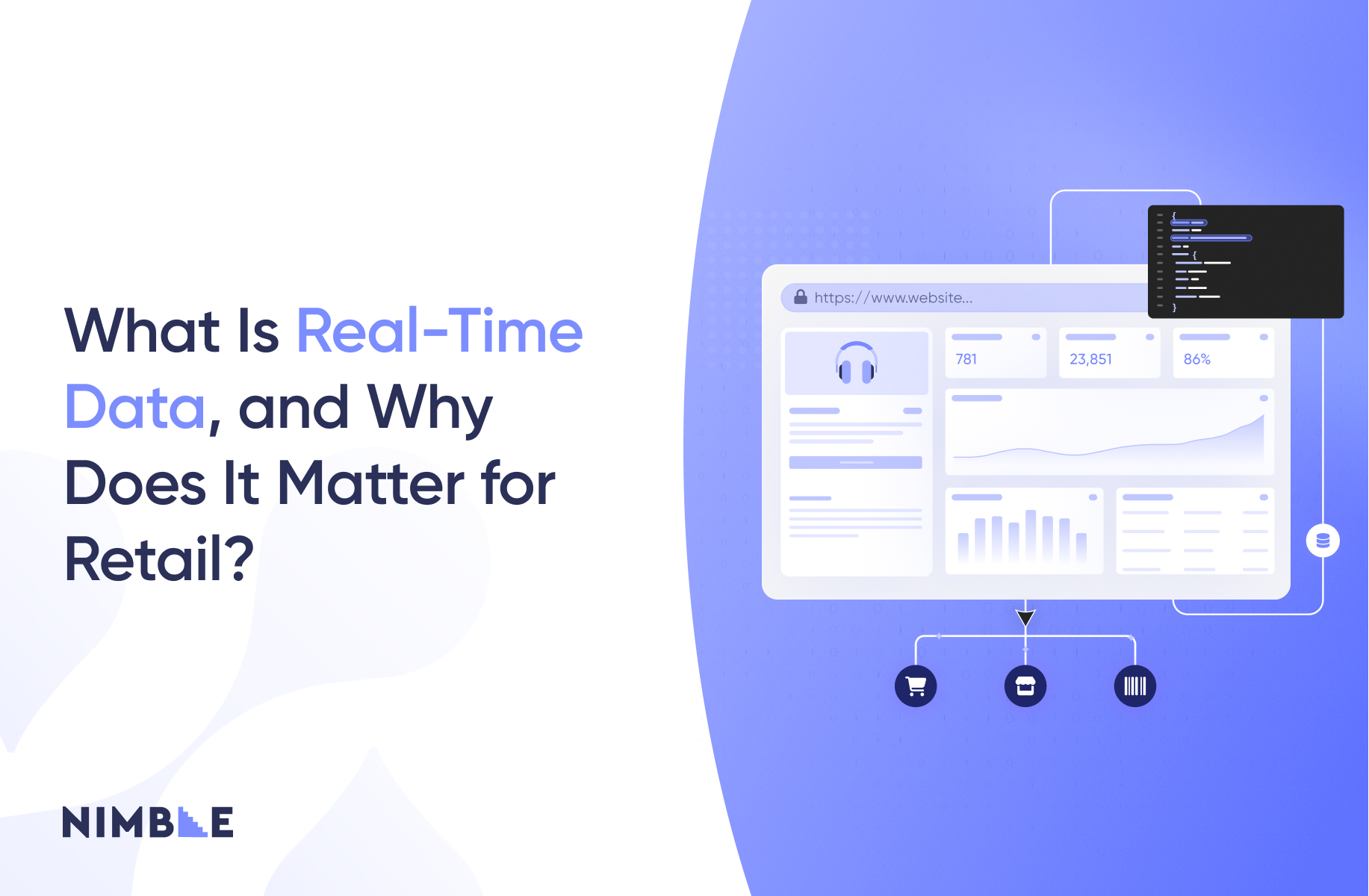
Veterans in the retail industry know success isn’t just about offering great products or competitive prices—it’s about timing. With consumer preferences, demand trends, and supply chain dynamics fluctuating and evolving more rapidly than ever, retail businesses need instant insights to maintain a competitive edge.
Integrating real-time data into a retail data analytics workflow allows businesses to act quickly on market changes and deliver personalized customer experiences, optimize inventory, enhance marketing, maintain competitive pricing, and transform many other aspects of retail operations.
Keep reading to learn how you can harness the power of real-time data to boost revenue and efficiency.
What Is Real-Time Data?
Real-time data is collected, processed, and made available as soon as it is generated. It is typically provided via data streams or pipelines that continuously collect data from live online sources such as social media, e-commerce websites, or specialized data intelligence platforms.
Unlike static or historical datasets which are delivered after the data is generated, real-time data provides immediate insights into the current state of the market, business operations, and performance, allowing retailers to act quickly and decisively.
How Real-Time Data Differs From Static Data
The Old Way: Static Data That Delivers Too Little, Too Late
Most retail businesses have traditionally relied on static datasets for all their data-fueled insights. Static data is recorded once and isn’t updated once it’s recorded. This provides “snapshots” that capture trends and behaviors during certain moments, but the data doesn’t dynamically adjust in real-time as new information becomes available. It’s also typically delivered with a delay of days or weeks.
While static data is adequate for identifying patterns and analyzing past trends to inform big-picture business strategies, it’s not very useful for adapting to quick fluctuations, like a sudden surge in customer demand for a specific product or a sudden weather event that delays restocking and influences competitor pricing.
The New Way: Real-Time Data That Provides Up-to-the-Second Holistic Insights
Real-time or dynamic data, on the other hand, operates like a live feed. It continuously updates to reflect current conditions, such as customer traffic in a store or the inventory levels of a trending product.
In retail, this difference matters. While static data might tell you what sold well last month, real-time data reveals what’s flying off the shelves right now—and right now is what retailers most need to know.
Batch Processing vs. Stream Processing
Real-time data often relies on stream processing (or “real-time processing”), which processes data as it is generated.
Stream Processing
As data flows into a data lakehouse, it is sorted and processed by automated data intelligence technology or, less commonly, by manual work of data engineers and analysts. After processing, the data flows into modeling and analysis workflows and technology. Processing occurs constantly to create a consistent “stream” of data.
Batch Processing
This approach contrasts with batch processing, where data (usually static) is collected over a period and then analyzed and processed in a scheduled “batch” later on. After the data is collected, it gets transferred to a processing workflow at a regularly scheduled interval, such as once a month, once a week, or once the dataset hits a certain size threshold.
To illustrate the difference, imagine a retailer running a flash sale. Batch processing would analyze the sale's impact hours later, while stream processing would show its effectiveness minute by minute.
Both approaches are valuable. However, when possible, stream processing is typically better for most modern data applications, especially in retail.
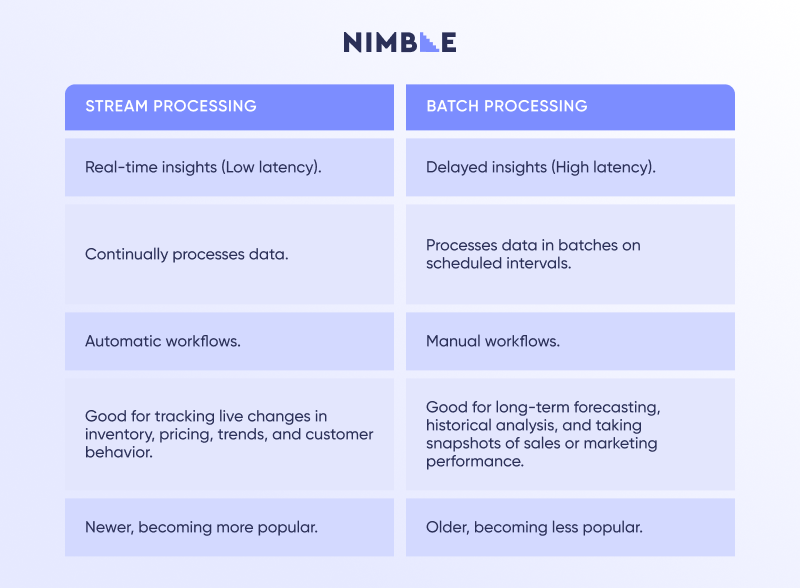
Why Real-Time Data Matters for Retail Decision-Making
Real-time data plays a critical role in providing the agility retailers need to respond quickly to shifts in trends, consumer demands, and supply chain factors. The most significant improvements real-time data enables include:
Enhanced Customer Experiences
By analyzing a customer’s browsing history, location, or purchase habits, retailers can offer personalized promotions, product recommendations, and in-store experiences. E-commerce stores can suggest complementary products to add to the cart before checkout, while brick-and-mortar stores can send tailored discounts to customers' phones as they browse specific aisles.
Better Operational Efficiency
Real-time data can help businesses identify and respond to bottlenecks and opportunities as they arise. For instance, live inventory tracking helps retailers restock popular items before they sell out, while dynamic workforce allocation ensures sufficient staffing during peak times.
Faster, Smarter Decision-Making
Retailers can use real-time data to respond quickly to abrupt changes in demand or trends. For instance, retailers could shut down promotions that aren’t working or increase prices in response to a sudden surge in demand.
4 Common Data Problems Real-Time Data Solves
A better way to contextualize how real-time data improves retail data analytics is to examine how real-time data solves problems the retail industry has traditionally experienced with static data.
Problem 1: Data Freshness
Problem: Data Latency = Inability to Keep Up With Dynamic Market Changes
Static data is always a step behind. By the time the data has been collected, processed, and analyzed, the market has moved on—often, retailers see data delays of 5 weeks or more. This makes it difficult to react quickly to social media trends, inventory disruptions, or competitor activity.
Solution: Instant Data = Ability to Adapt Quickly
Real-time data allows retailers to constantly monitor and instantly gain insights into any factor that might influence their business. With real-time data, retailers can respond immediately to shifts in customer demand, trends, competitor pricing adjustments, or external events like weather or political news.
Problem 2: Limited Context and Flexibility
Problem: Limited Context and Scope = Incomplete View of the Market
Static datasets typically focus on narrow slices of information, such as inventory levels, sales figures, or pricing trends, and only deliver insights on an intermittent basis. Traditional data dashboards are also limited in their ability to quickly add or remove data sources or adjust parameters. This leaves retailers guessing how external factors like regional economic shifts, competitor actions, or customer sentiment might influence their data.
Solution: Full Context & Flexible Scope = Comprehensive View of the Market
Real-time data solutions often aggregate information from multiple sources, such as pricing platforms, social media, weather reports, and customer feedback. Data from all these sources can be integrated and delivered in unison via the same solution, allowing retailers to see the complete market picture and adjust their strategies in real-time. Real-time data is also more flexible, allowing businesses to refine data inputs or adjust granularity.
Problem 3: Managing Many Different Vendors & Datasets
Problem: Combining Dozens of Independent Datasets = Fragmented Decision-Making
Most retailers juggle dozens, if not hundreds, of independent datasets sourced from different systems and vendors. These siloed datasets create a fragmented picture of the business, making it hard for teams to align their decisions and optimize efficiency.
Solution: One Streamlined Data Source = Aligned Decision-Making
By consolidating data into a single real-time stream, retailers eliminate siloes and foster collaboration across teams. Marketing, inventory, and operations teams can all work from the same up-to-date dataset or collection of datasets, ensuring alignment and reducing miscommunication. This unified approach supports faster, more consistent decision-making.
Problem 4: Capped Efficiency
Problem: Manual Processes = Inefficient and Costly
Static data typically requires extensive manual processing, from cleaning and integrating datasets to running analyses. This work consumes valuable time and resources, leaving data teams bogged down in rote operational tasks instead of focusing on more strategic initiatives.
Solution: Automated Processes = Better Efficiency and Strategic Focus
Real-time data eliminates much of the manual work associated with static datasets. Automation handles tasks like data cleaning, integration, and analysis, freeing up data teams to focus on strategic initiatives that drive growth. Retailers benefit from faster insights, fewer errors, and more efficient operations.
How Retailers Are Leveraging Real-Time Data
Retailers worldwide are embracing real-time data to enhance operations, improve customer satisfaction, and increase revenue and success. Here are a few specific ways retailers can use real-time data to inform important business decisions.
Driving Dynamic Competitive Pricing
Real-time data enables retailers to quickly adjust prices or automatically set dynamic pricing based on fluctuating factors like:
- Competitor Pricing: Retailers can monitor competitor prices, discounts, and promotions in real time and adjust their own prices to remain competitive, driving higher sales volumes without sacrificing profit margins.
- Inventory Levels: By analyzing inventory data like stock levels across stores or on competitor sites, businesses can implement strategies like markdowns for slow-moving products or price increases for fast-selling items to optimize turnover.
- Market Trends: By collecting real-time updates on social trends, news events, customer sentiment, or global pricing variations, retailers can anticipate demand surges and price their products accordingly, capturing maximum value during peak periods.
Example
A fashion retailer notices it is rapidly losing market share on a specific sundress to competitors with lower pricing. The retailer uses real-time competitor pricing data to implement a dynamic pricing system that automatically adjusts the sundress’s price to ensure it is always $1.00 lower than competitors' prices. This raises sales of the dress by 30%.
Measurable Benefits
- Increased Revenue: Optimizing prices to capture demand at the right moment.
- Improved Competitiveness: Staying aligned with or ahead of market trends.
Digital Shelf Optimization
Digital shelf optimization ensures a retailer’s product listings are always presented in the best way to drive clicks and conversions. Real-time data enables retailers to:
- Adjust Product Placement: Data from search and category pages helps retailers fine-tune the order in which products appear, prioritizing bestsellers or items with high profit margins.
- Enhance Descriptions and Imagery: Monitoring which keywords, images, or product descriptions resonate with customers allows retailers to optimize listings on the fly.
- Track Competitor Content: Analyzing how competitors describe similar products provides actionable insights for improving one’s own digital shelf.
Example
A retailer identifies a product trending on social media but notices it’s buried on the fifth page of their e-commerce search results. By prioritizing its placement on the homepage and improving its description, they increase sales by 20%.
Measurable Benefits
- Higher Conversion Rates: Optimized product listings attract more clicks and purchases.
- Enhanced Customer Satisfaction: Easy navigation and relevant products create a better shopping experience.
Customer Listening and Sentiment Analysis
Understanding how customer sentiment changes in real-time is a game-changer for retailers. By analyzing reviews, ratings, and social media activity, they can uncover insights into customer pain points, preferences, and emerging trends. Real-time sentiment analysis helps retailers to:
- Influence Product Design: Insights from customer feedback can guide product improvements or the creation of entirely new offerings tailored to consumer demand.
- Get Ahead of a Crisis: Real-time monitoring of negative sentiment can alert teams to potential issues, enabling swift action to resolve problems and preserve brand reputation.
- Spot Emerging Trends: Social media buzz and online discussions can highlight upcoming trends, helping retailers adapt their product mix before the competition.
Example
A retailer notices an influx of negative reviews about a product's durability. By redesigning the product and launching a marketing campaign emphasizing the improvement, they win back customer trust and increase sales.
Measurable Benefits
- Improved Customer Experience: Addressing issues before they escalate enhances loyalty.
- Proactive Crisis Management: Spotting and resolving problems early prevents damage to the brand's reputation.
- Data-Informed Innovation: Feedback informs better product development and targeted marketing.
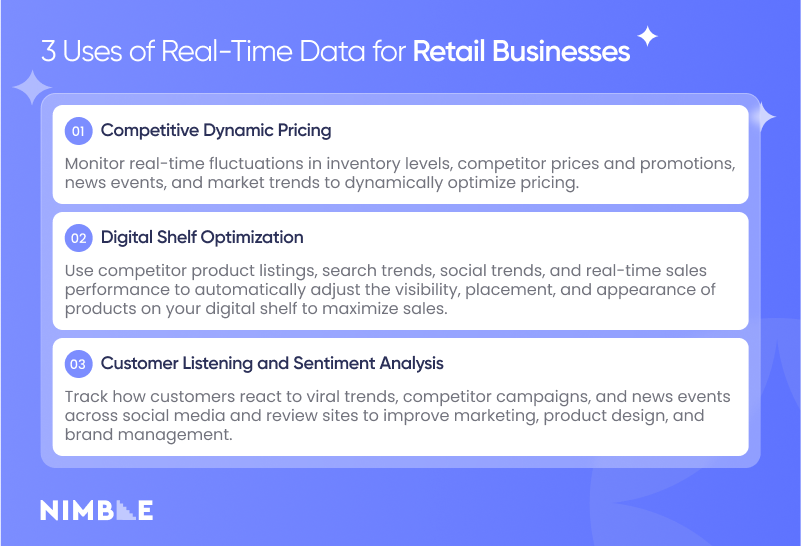
How Nimble’s Online Pipelines Make Real-Time Data Easy for Retailers
Nimble’s Online Pipelines is a comprehensive real-time data solution that delivers granular, accurate, and fully processed data from dozens of relevant retail and e-commerce data sources, making integrating real-time data analytics into your business easier than ever.
Our online pipelines deliver:
- Fast Data Processing: Real-time insights are available in seconds with our AI-powered data cleaning and processing systems.
- Easy Implementation: Plug-and-play pipelines connect effortlessly with existing systems and popular data workflows like Databricks.
- Reliable Accuracy: Dependable data ensures confident decision-making.
- Effortless Flexibility: Choose from dozens of relevant data sources, including e-commerce platforms, social media, SERP data, and map data.
- Integration with AI: Our retail online pipelines are built to be compatible with AI and machine learning systems.
After integrating online pipelines into their data workflows, retail companies have achieved a 10x improvement in data accuracy and a 45% increase in lead generation.
Conclusion: Power Your Retail Strategy with Real-Time Data from Nimble
In the modern retail landscape, real-time data isn’t just nice to have—it’s absolutely necessary to remain competitive.
From enhancing customer experiences to optimizing inventory and enabling dynamic pricing, having access to accurate, comprehensive data that adjusts dynamically is essential to your business strategy.
Nimble’s real-time Online Data Pipelines make integrating real-time data analytics simple for retailers. Don’t let outdated processes hold you back—empower your business with instant insights that drive smarter decisions and better outcomes.
Unlock instant real-time retail insights with Nimble. Talk to us to learn more.
FAQ
Answers to frequently asked questions