Why Sentiment Analysis is the Missing Link in Your Retail/E-commerce VoC Strategy
Discover how adding AI-powered customer sentiment analysis to your VoC strategy can enable better marketing, product, and operational decisions.


Every good retail or CPG business knows an effective voice of the customer (VoC) strategy demands a deep, accurate, and nuanced understanding of your customer’s emotions.
However, despite the wealth of customer feedback data generated from online reviews, social media, and customer service interactions, transforming this data into actionable insights is challenging, time-consuming, and sometimes downright impossible. This leads to missed opportunities to improve customer experiences, foster loyalty, and optimize product offerings.
Fortunately, AI-powered customer sentiment analysis technology can make the process easier, faster, and more effective. Keep reading to learn how brands can use it to transform customer emotions into actionable business insights at the speed and scale of the modern market.
What is Sentiment Analysis, and How Does It Work?
What Is Sentiment Analysis?
Sentiment analysis, sometimes called opinion mining, is the process of interpreting text, video, or audio to identify and categorize the emotions and attitudes conveyed by the content creator, typically using advanced AI technologies.
Basic sentiment analysis models categorize sentiment as positive, neutral, or negative. More advanced models can recognize specific emotions, like anger or happiness, and distinguish between different topics.
What Is Customer Sentiment Analysis?
Customer sentiment analysis uses sentiment analysis technology to analyze and categorize customer feedback, with the intent of using it to drive better product, marketing, and business decisions.
Essentially, customer sentiment analysis transforms a chaotic sea of customer feedback into a neatly organized, color-coded map, where each shade represents a specific emotion or opinion.
How Technology Enables Customer Sentiment Analysis
Sentiment analysis relies on AI and scalable data-gathering technology to transform raw, unstructured data into categorized, understandable insights.
After raw data is gathered and processed, natural language processing (NLP) algorithms break down text into smaller, analyzeable components, such as words, phrases, and syntax, to interpret meaning and context. Computer vision technology can do the same for images and video. Then, machine learning models analyze patterns and trends within the data to refine predictions over time.
Here’s a breakdown of the process:
- Data Collection: The system gathers input from multiple channels, such as product reviews, chat transcripts, emails, or social media posts.
- Preprocessing: The data is cleaned and standardized to remove noise, such as irrelevant text or repeated entries.
- Analysis and Classification: Algorithms categorize the sentiment of each input as positive, negative, or neutral. More sophisticated systems identify specific emotions like frustration, joy, anger, or indifference, and some can even tackle nuanced expressions like sarcasm or mixed sentiments.
- Insight Extraction: Notable trends, such as recurring complaints about delivery times or praise for product quality, are highlighted and presented using answer agents or regular retail data analytics tools.
Why Customer Sentiment Analysis Matters for Retail
Customer sentiment analysis allows retailers to better understand their customers' needs, wants, and pain points, enabling a more effective voice-of-customer strategy.
By using technology to evaluate and analyze customer feedback across all important feedback channels, retailers can understand how customers perceive their products, services, and overall brand image. Retailers can uncover widespread dissatisfaction with a product feature or an opportunity to build on a popular trend, allowing them to improve product design, customer service, marketing campaigns, operations, and more.
In essence, sentiment analysis isn’t just about categorizing feedback—it’s about enabling retailers to take meaningful, data-driven actions that improve customer satisfaction, loyalty, and profitability.
5 Ways Customer Sentiment Analysis Can Improve Retail Business Operations
Well–implemented customer sentiment analysis can allow retailers to gain a more holistic, accurate, and up-to-date understanding of their customer’s opinions. These opinions can be leveraged to make more effective (and faster) marketing, product, and operational decisions. Here are 5 areas sentiment analysis can impact the most.
Improved Customer Experience
Understanding patterns and trends in customer sentiment allows retailers to improve and personalize customer service interactions, website user experience, and other customer-facing aspects of their business.
For example, detecting patterns of frustration in feedback, such as repeat issues with customer support response time, allows customer support teams to tackle the root cause of the problem by adding better time-tracking to their ticketing system. This prevents the issue from happening again, transforming future negative experiences into positive ones.
Similarly, tracking patterns in positive sentiment—such as enthusiastic reviews on a specific product line—provides opportunities to upsell complementary products or foster deeper engagement through loyalty programs.
Stronger Brand Loyalty
Proactively addressing negative feedback demonstrates that a brand truly listens to its customers. This builds a foundation of trust that enables long-term loyalty, less churn, and more repeat business.
For example, sentiment analysis can detect repeat issues multiple customers are having with delayed shipping or defective products. By identifying these patterns and fixing them (rather than treating them as isolated incidents), retailers can appear more attentive, professional, and caring toward their customers.
Positive engagement strategies, such as finding and publicly acknowledging instances where customers are satisfied, can also strengthen emotional connections with the brand.
Better-Informed Product Decisions
Customer sentiment insights are invaluable for refining product development. If reviews consistently highlight dissatisfaction with a product’s durability or design, brands can prioritize updates to address these concerns, aligning offerings more closely with customer desires.
Additionally, positive trends—like praise for specific features—can inform future product lines. These insights also allow retailers to stay ahead of competitors by quickly adapting to changing customer needs.
Identifying Areas of Improvement
Sentiment analysis helps retailers pinpoint weaknesses in their operations, whether they involve product quality, customer service, or website navigation.
By continuously monitoring customer feedback, retailers can identify recurring issues and proactively address them. This ensures that businesses are not only reacting to complaints but are also preventing potential negative experiences in the future.
Tailoring Marketing Campaigns
By analyzing sentiment around specific products, campaigns, or events, retailers can adjust their marketing messages to resonate more effectively with customers.
Positive sentiment may encourage the amplification of successful campaigns, while negative sentiment can signal the need for a more targeted approach or changes to the messaging. Tailoring campaigns based on sentiment data allows brands to maximize engagement and optimize their marketing spend.
How to Leverage Customer Sentiment Analysis in Your VoC Strategy in 4 Steps
So, how exactly do you integrate customer sentiment analysis into your retail voice of the customer strategy? Here’s a quick step-by-step guide.
Step 1: Collect Data from the Right Channels
Start by gathering data from every channel your customers are leaving feedback in. This typically includes:
- Product reviews (on your site and general review sites)
- Social media platforms (posts, comments, videos, etc.)
- Email surveys
- Customer service interactions (support tickets, emails, etc)
To maximize effectiveness, ensure the data is recent and representative of your target audience. Consider integrating multiple touchpoints to capture a comprehensive view of customer sentiment across the buyer journey and implementing data solutions with real-time gathering capabilities.
Step 2: Analyze Sentiment with the Right Tools
Use sentiment analysis tools that utilize AI and NLP algorithms to detect trends, categorize emotions, and pinpoint actionable insights. Ensure your tools can handle data at the scale your business needs and have the capacity to handle advanced insights, like identifying recurring issues in product reviews or emerging trends in social conversations.
This step can be tricky because these sentiment analysis tools are generally built-in to data processing or retail data analytic platforms. Some independent sentiment analysis tools include:
A full list is available here.
Step 3: Take Action on Insights
Use the insights gained to:
- Address negative sentiment promptly, offering solutions that demonstrate empathy and a commitment to improvement.
- Amplify positive customer stories in marketing campaigns to build brand credibility and attract new customers.
- Enhance products and services based on customer feedback, such as refining designs, adjusting pricing, or improving delivery experiences.
Engaging cross-functional teams, like marketing, product development, and customer support, ensures these actions are implemented effectively.
Step 4: Monitor Results for Continuous Improvement
Track KPIs such as net promoter score (NPS), retention rates, sales, and customer effort scores (CES) to measure the impact of your sentiment-based strategies.
Regularly review these metrics to identify trends and adapt your approach accordingly. For example, if NPS improves after addressing a common complaint, investigate how to replicate that success in other areas. Continuous monitoring and adjustment will help you stay aligned with evolving customer expectations.
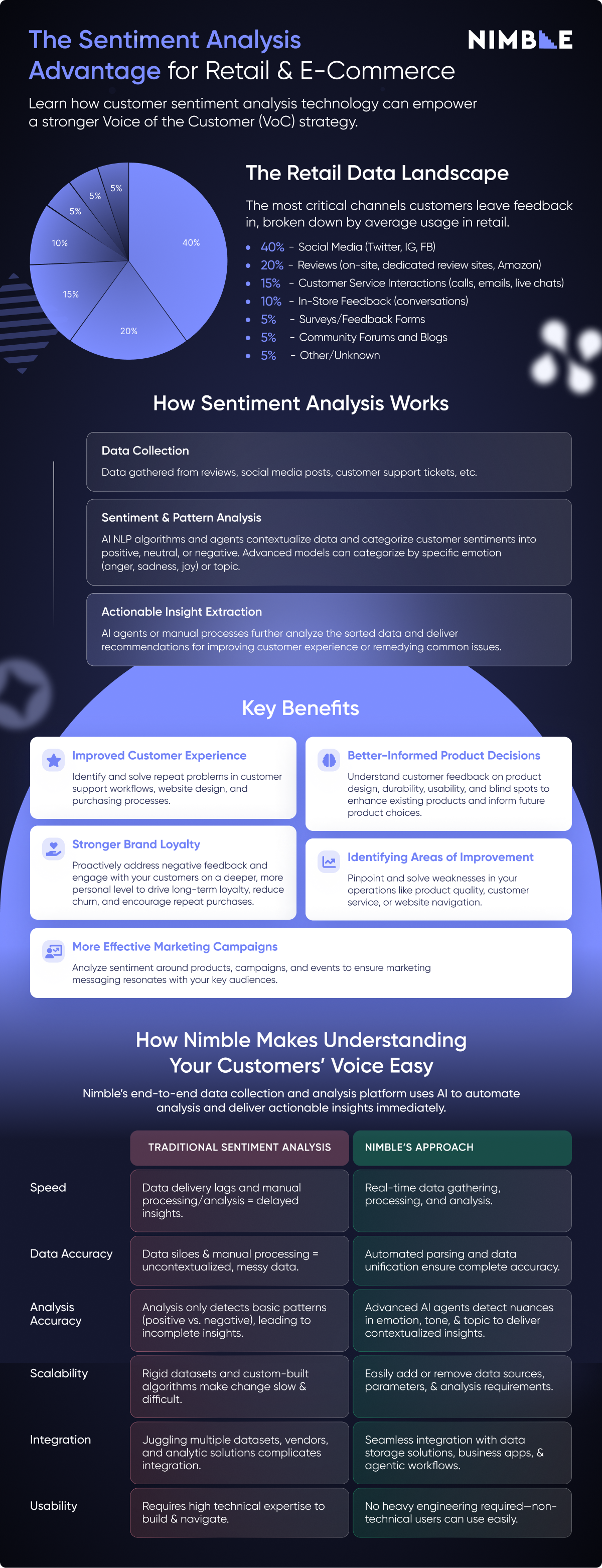
Common Challenges in Retail Sentiment Analysis (and How to Overcome Them)
Challenge 1: Data Overload
The Problem
Retailers face an overwhelming amount of customer feedback data in diverse formats from multiple sources. Product reviews, social media posts, forum comments, email surveys, support tickets, and even in-person interactions all contain tons of data that needs to be analyzed. This data deluge often leads to analysis paralysis, where the sheer volume of information makes it difficult to identify what's truly important.
The Solution
Having a unified data solution that can take in and process data from multiple sources can offer a huge edge in making data more manageable. Keeping your data storage and data processing workflow organized, like by using medallion architecture in data lakehouses, also helps.
But the most important thing is to utilize automated data processing and advanced AI tools that can sift through data, remove irrelevant noise, and highlight key insights in a timely manner for you.
Challenge 2: Unstructured Data Chaos
The Problem
Customer feedback frequently comes in diverse formats—think free-form text, emojis, and even audio or video reviews. This lack of structure makes it challenging to process and analyze the data effectively.
The Solution
Using a data gathering and processing tool that can integrate seamlessly with various data sources and handle a wide variety of data diversity is key to containing chaos. For example, Nimble’s Online Knowledge Cloud can gather any data type from any source, and utilizes AI tools to normalize this data into structured formats. This enables deeper analysis and consistent insights across channels.
Challenge 3: Technical Complexity
The Problem
Sentiment analysis solutions are powered by highly specific AI technologies that require advanced technical expertise and significant setup time to implement. This leaves small teams or non-technical staff struggling to leverage this powerful customer voice tool.
The Solution
Aside from putting together your own team of AI and data engineers, the most simple solution is to use an end-to-end data platform that handles data gathering, processing, and sentiment analysis and integrates easily into your existing customer voice workflow. Nimble’s Online Knowledge Cloud is built for retail sentiment analysis and is ready to process and analyze data from every relevant channel without the need for complex engineering.
Challenge 4: Turning Data into Action
Problem
Raw data by itself is useless—it must be cleaned, processed, contextualized, and analyzed to create insights. But even then, the quality and depth of those insights matter. Many sentiment analysis tools simply identify patterns, leaving retailers struggling to translate sentiment analysis into tangible actions that impact their bottom line.
Solution
Every step of your sentiment analysis process needs the most advanced technology to ensure the best performance. Raw data must come from only relevant sources in real-time. Cleaning and processing workflows must be quick and ensure accuracy. AI agents and sentiment analysis technology must be effective at detecting specific emotions, sarcasm, and other subtle variations. And finally, it helps if you’re using a solution that uses LLMs to contextualize analyzed data and generate actionable insights for you.
Conclusion: Refine Understanding of Your Customers’ Voice With Nimble
Understanding customer sentiment is a critical piece of the Voice of the Customer puzzle for retailers who aim to stay competitive in today’s market. Luckily, in today’s world, advancements in AI and data technology are making it easier to understand your customers’ wants, needs, and problems on a massive scale.
If you’re looking to implement advanced sentiment analysis into your VoC strategy, Nimble’s Online Pipelines and Online Knowledge Cloud enable brands to gather, analyze, and act on sentiment data faster than ever.
By leveraging AI-powered data contextualization; search, semantic, and answer agents; automated data parsing, and sentiment analysis algorithms, Nimble helps retailers detect sentiments and key themes from text, images, and even audio data.
Ready to decode your customers’ emotions and boost loyalty? Get in touch with our team.
FAQ
Answers to frequently asked questions