How to Reduce Revenue Loss with Data-Driven Retail Insights
Get actionable advice on turning real-time data into revenue protection, from pricing and inventory to promotions and forecasting.
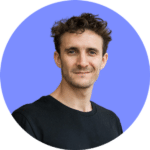

In retail, margins are thin, competition is fierce, and even minor missteps can cost millions. Revenue loss from preventable issues like pricing errors, overstock, and missed demand signals continues to plague the industry. But forward-thinking retailers are solving these problems with one thing: real-time, data-driven retail insights.
With today’s technology, retailers can turn fragmented data into a strategic asset that prevents stockouts, fine-tunes pricing, and captures more revenue.
This article breaks down how you can use real-time analytics to plug revenue leaks and future-proof your operations. Here are some of the topics it covers:
Key Takeaways
- Revenue loss in retail is often preventable: Common culprits include pricing mistakes, inventory mismanagement, and ineffective promotions.
- Real-time, data-driven insights help retailers stay ahead by enabling dynamic pricing, smarter forecasting, and optimized stock allocation.
- Predictive analytics and automation empower teams to move from reactive firefighting to proactive planning.
- Connected, fresh data across systems ensures better decision-making and minimizes costly blind spots.
What Causes Revenue Loss in Retail?
Revenue loss in retail can be caused by many different factors, but the most common are pricing mistakes, stockouts & overstock issues, and ineffective promotional strategies. We’ll break down each of these below.
Common Pricing Mistakes
Pricing is one of the most sensitive levers in retail profitability. Even small errors can erode margins, drive away customers, or trigger unnecessary competition. Some of the most common pricing pitfalls include:
- Mispricing due to poor data visibility: Many retailers rely on static pricing models or outdated competitor data, leading to prices that don’t align with customer expectations or market conditions. This makes it hard to identify the optimal pricing window.
- Markdown errors and margin erosion: Excessive markdowns often result from improper demand forecasting. Steep discounts offered too soon can slash margins. But delaying them can lead to dead stock that clogs cash flow.
- Price wars and the race to the bottom: Competing on price alone can be a dangerous game. Blindly matching or undercutting competitors erodes profitability. A better approach is dynamic pricing that considers competitor and consumer behavior.
Stockouts and Overstock Issues
Inventory mismanagement is one of the leading causes of revenue loss in retail. When demand fluctuations are not accounted for, retailers either miss out on sales due to stockouts or tie up capital in excess inventory.
- Stockouts drive customers elsewhere, often leading to permanent loss of loyalty. Worse, stockouts disrupt omnichannel shopping experiences, frustrating customers who expect seamless availability online and in-store.
- Overstock leads to dead inventory, which takes up storage space and forces heavy discounting just to clear shelves. Fashion and seasonal retailers are particularly vulnerable, as trends shift rapidly.
- Lack of demand forecasting: Without real-time data on buying trends and broader market shifts, retailers are left guessing how much stock they need.
Ineffective Promotional Strategies
Running promotions without data can backfire. If a discount is too steep, or it’s offered at the wrong time, it can lower revenue and cannibalize full-price sales. Many retailers fall into this trap because they lack the tools to evaluate promotion performance. Without access to predictive analytics in retail, they can’t anticipate consumer response and have to resort to educated guesswork.
When promotional strategies are not based on historical patterns and actual retail consumer insights, the effects can be damaging:
- Discounts can erode perceived value, particularly if they’re steep and frequent. This trains customers to wait for sales. This delay in purchasing can sabotage long-term revenue.
- Blanket discounts that lack personalization can lead to wasted marketing spend due to a lack of proper customer segmentation. For example, consider a store offering a 20% discount to a customer who was already willing to buy at full price. This reduces revenue unnecessarily.
- Failure to measure promotional effectiveness: Many retailers launch promotions without tracking their impact in real time. Well-structured retail analytics data can help retailers understand which promotions actually drive incremental revenue rather than just shifting purchases forward.
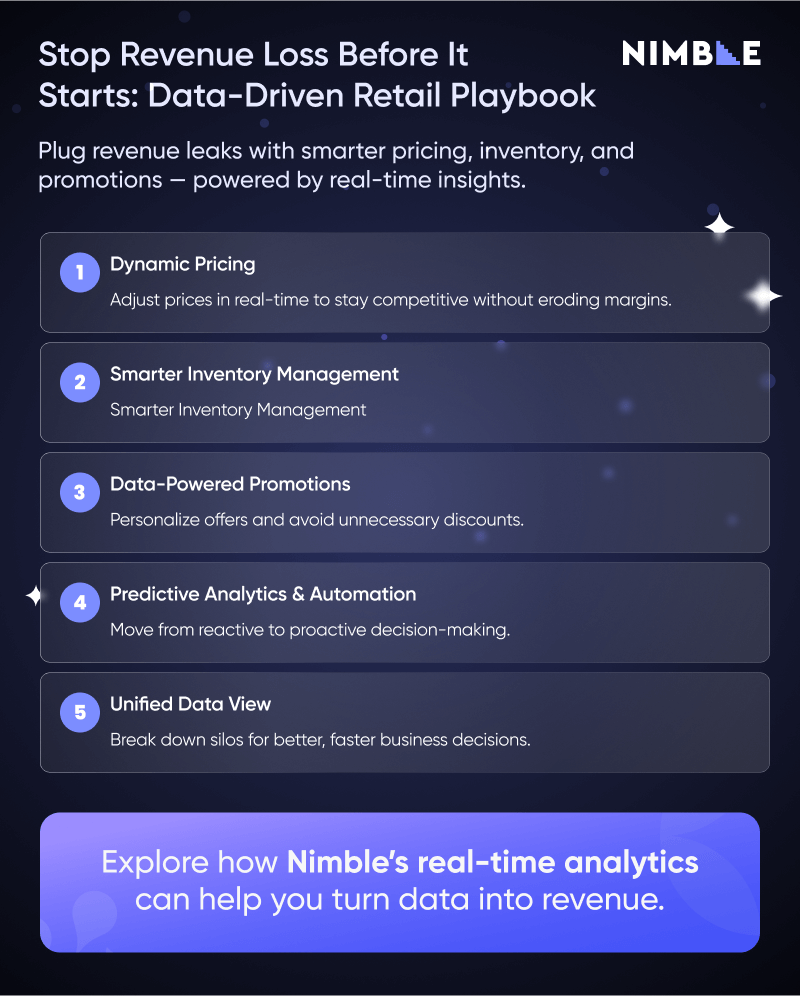
How to Use Data-Driven Retail Insights for Revenue Loss Prevention
Real-time data analytics prevents revenue loss at every stage of the retail lifecycle, from demand forecasting and pricing to inventory allocation and even post-sale optimization.
Here’s how having a steady, reliable stream of up-to-date information makes all the difference in an environment where timing is everything:
Real-Time Data for Smarter Pricing
Dynamic pricing models use real-time data inputs to adjust pricing in the moment. These inputs include factors like competitor prices, demand shifts, and inventory status. Instead of fixed discount schedules or reactive markdowns, retailers can optimize margins while staying competitive.
For example, a brand selling on multiple marketplaces can use real-time pricing data to respond instantly to undercutting by a competitor, avoiding the race to the bottom.
Use Case Spotlight: A large home goods brand uses Nimble’s Online Pipelines to monitor pricing shifts on Amazon and Target. When a competitor drops prices, their system triggers an instant price match for specific SKUs. This preserves competitiveness without sacrificing margins across the board.
Inventory Analytics for Stock Optimization
Predictive analytics powered by machine learning helps retailers strike the right balance between too much and too little inventory. These models incorporate sales history, seasonal demand, competitor launches, and even weather to forecast accurately.
Real-time visibility into stock levels and performance across channels enables better allocation and smarter reorder decisions. These help to minimize overstock and reduce the risk of sellouts.
Use Case Spotlight: A grocery chain uses AI-powered inventory alerts to adjust product replenishment based on regional demand. During a heatwave, sales of beverages spiked. As a result, predictive analytics triggered a stock transfer that helped avoid shelf-outs.
AI and Automation in Retail Decision-Making
AI helps retailers move from reactive to proactive. From detecting pricing anomalies to triggering stock alerts and automating promotions, AI systems reduce manual effort and improve precision, driving measurable gains in inventory accuracy and operational efficiency across major retail networks. Automating routine tasks like price checks and inventory adjustments also frees up staff to focus on higher-impact activities, such as customer service and visual merchandising.
Nimble’s Knowledge Cloud streams real-time retail data directly into business intelligence tools, powering automated decision-making across pricing, inventory, and more.
Explore Nimble’s solutions for real-time retail analytics.
3 Best Practices for Implementing Data-Driven Analytics in Retail
Successfully leveraging data isn’t just about collecting more of it. It’s about capturing the right data, interpreting it accurately, and ensuring it flows freely across systems. Just as important is making sure that data is fresh, because yesterday’s insights can’t solve today’s challenges.
Retailers that master the following three practices can anticipate shifts, act faster, and significantly reduce revenue leakage.
1. Choosing the Right Data Sources
Your data strategy is only as strong as the inputs that feed it. To create a full picture of what’s happening—and what’s about to happen—retailers need to combine multiple data streams:
Point-of-Sale (POS) Data
POS systems provide granular retail category insights into what’s selling, where, and when. This data is foundational for understanding purchase patterns and managing inventory more effectively. Advanced systems can segment POS data by store location, SKU, time of day, and even associate performance. Retailers rely on this to optimize everything from product placement to staffing.
Customer Behavior Data
E-commerce platforms, loyalty programs, and in-store footfall trackers are gold mines of behavioral insight. Analyzing session durations, bounce rates, cart abandonment, and purchase history helps tailor marketing strategies and optimize merchandising. For example, identifying a customer’s affinity for a product category or price range can power personalized offers that drive higher conversion rates.
Competitor and Market Data
Real-time competitor price tracking and market benchmarking tools allow retailers to adapt pricing dynamically. This prevents margin erosion while staying competitive. Web scraping solutions and third-party data providers can help you stay on top of market shifts, trending products, and promotional cadences across your segment.
2. Using Predictive Analytics for Forecasting
Once you capture the right data, predictive analytics transforms it into foresight. Retailers can shift from reactive to proactive by forecasting trends, inventory needs, and customer demand.
Demand Forecasting Models
Advanced machine learning models ingest historical sales data, seasonality, external factors (like weather or holidays), and real-time buying trends to predict future demand at the SKU level. This allows for more precise stock planning and promotional timing, reducing both stockouts and overstock scenarios.
Customer Churn & Lifetime Value Prediction
Predictive models can flag customers likely to churn or estimate their lifetime value. Retailers can act early enough to make a difference. For example, they may trigger a retention campaign for a high-value customer who hasn't purchased recently, or is showing declining engagement.
Price Elasticity Modeling
Predictive analytics can also estimate how sensitive certain products or customer segments are to price changes. This enables smarter markdown planning and avoids discounting products that will sell at full price.
3. Avoiding Data Silos for Better Decision-Making
Data is only valuable if it’s accessible across the organization. Unfortunately, many retailers suffer from siloed and fragmented systems that prevent departments from seeing the full picture.
Unified Data Infrastructure
Integrating POS systems, CRM platforms, inventory tools, and e-commerce data into a single platform (like a retail data lake or real-time analytics stack) is essential. This eliminates version conflicts and enables cross-functional insights. For example, combining inventory levels with real-time sales and marketing campaign performance can help dynamically shift stock between regions.
Real-Time Data Pipelines
Batch reporting has its place, but it’s often too slow for modern retail. Real-time data pipelines powered by event streaming tools (like Kafka or Flink) let retailers monitor key metrics continuously. This enables instant reactions to stockouts, pricing anomalies, or unexpected demand spikes.
Cross-Functional Dashboards
Visualizing unified data in intuitive dashboards ensures that executives, merchandisers, marketers, and store managers are in full alignment, always. It also empowers on-the-ground staff with insights they can act on, like restocking fast-moving items, or adjusting pricing for localized promotions.
Stop Revenue Loss Before It Starts With Nimble’s Real-Time Insights
In an industry where timing, precision, and agility define success, real-time data is a critical line of defense against revenue loss. From preventing stockouts and optimizing pricing to making smarter promotions and forecasts, the retailers who win are those who can anticipate and respond to trends proactively.
By implementing connected, real-time data strategies across your retail operations, you can move faster, minimize costly errors, and make decisions that grow your bottom line instead of draining it.
Now’s the time to stop guessing and start leveraging data-driven decision-making. Explore Nimble’s solutions for real-time retail analytics to see how your data can work harder for you.
FAQ
Answers to frequently asked questions