The Importance of Data Parsing for Making Smarter Business Decisions
Learn why data parsing is essential for fueling business strategies in retail, finance, real estate, and AI.

.png)
The web is full of more valuable data than ever before: customer comments on social media, promotions and product updates from competitor websites, live asset prices—but without data parsing, there’s no way to make use of it.
Data collected from the web typically arrives in a raw, unstructured format, making it difficult to analyze and extract actionable insights. Data parsing acts as a crucial step in data processing by converting this raw data into organized, structured formats that businesses can use for analysis, decision-making, automation, and optimizing business operations.
In this blog, we’ll explore why parsing unstructured data is essential for fueling smarter, more effective data-driven decisions in the retail & e-commerce, finance, real estate, and AI sectors.
Key Takeaways
- Data parsing is essential for transforming raw data into usable data that can fuel better business decisions, efficiency, and market insights.
- Structured data is required to prevent low-quality data and power important automation and business intelligence.
- Data parsing improves operations in retail/e-commerce, finance, real estate, and AI/machine learning.
- Automation plays a crucial role in scalable, efficient data parsing.
Want to know more about what data parsing is and how it works? Read A Comprehensive Guide to Data Parsing: What It Is and Why It Matters.
Why Businesses Need Data Parsing
Modern businesses need data to fuel everything from big-picture marketing, product, and staffing decisions to granular campaign, operations, or pricing tweaks. Data forms the foundation of modern business intelligence, and data parsing is a necessary step in making this data useful.
Without clean and structured data, organizations can’t make informed, data-driven business decisions, boost operational efficiency, enable real-time insights, or respond to customer demand.
Why You Can’t Use Unstructured Data
When businesses collect raw data from social media, customer support logs, documents, or any type of website, it usually arrives in an unstructured format—meaning it’s a mess of unformatted text, images, and numbers without any organization. This makes it difficult to analyze without first processing it.
7 Common Issues With Unstructured Data
Businesses must either process unstructured data to make it usable or use it as-is and risk a myriad of issues. The following are some of the problems with unstructured data:
1. High Volume
Businesses deal with overwhelming amounts of data from diverse sources, such as social media, customer reviews, emails, and third-party platforms. Without a scalable data parsing solution, analyzing this vast data becomes impossible, leading to missed insights.
2. Poor Data Quality
Without parsing, businesses struggle to identify and correct errors in unstructured data. Incomplete, duplicate, or outdated information leads to poor decision-making and unreliable analytics.
3. Inconsistency
Data often comes in different, inconsistent formats—from PDFs and images to raw text and Excel sheets. This prevents businesses from consolidating data into a unified view, resulting in fragmented or misleading analysis.
4. Lack of Organization
Unstructured data lacks clear labeling and categorization, making it challenging to query or extract relevant insights.
5. Automation Gaps & Workflow Bottlenecks
Automated processes require clean, structured data. When businesses work with unstructured data, they often resort to manual data entry or error-prone scripts. This slows operations and increases the risk of inaccuracies.
6. Storage Inefficiencies
Storing unstructured data without parsing leads to bloated databases and inefficient storage use. Structured data is easier to manage, reducing storage costs and improving data retrieval speeds.
7. Integration Challenges
Businesses often integrate data from multiple sources, such as CRM systems, e-commerce platforms, and third-party APIs. Unstructured data complicates this integration and limits the ability to create a comprehensive data ecosystem.
The Impact of Poor Data on Business Decision-Making
If businesses fail to process their unstructured data effectively, they end up fueling important analytics platforms, automations, and data analysis workflows with inaccurate, messy, and stale data.
Poor data quality has far-reaching effects that can lead to serious impacts on revenue and performance, including:
Misleading Insights
Faulty data skews reports and dashboards, leading to decisions based on incorrect assumptions. Marketing teams may target the wrong audience, or finance teams may miscalculate revenue projections, resulting in wasted budgets and missed growth opportunities.
Missed Opportunities
Without reliable data, businesses fail to recognize emerging trends or market shifts. Competitors with access to accurate, parsed data can respond quickly to market demands, launch new products, or adjust pricing strategies while others lag behind.
Resource Drain
Data scientists, analysts, and IT staff often waste hours troubleshooting errors instead of focusing on generating actionable insights, leading to productivity losses.
Compliance Risks
Regulatory bodies, especially in industries like finance, demand precise and timely reporting. Poor data management can result in non-compliance, leading to hefty fines, reputational damage, and legal issues.
Inaccurate Forecasting
Predictive models and AI systems rely on clean, structured data to generate accurate forecasts. Poor data leads to unreliable predictions, impacting inventory planning, revenue forecasts, and supply chain management. Businesses may overproduce, understock, or miss sales targets.
Customer Dissatisfaction
Incomplete or incorrect data about customer preferences, purchase history, or interactions can result in erroneous personalization attempts or failed product recommendations. This can damage brand loyalty and increase churn rates.
Delayed Decision-Making & Lack of Agility
When leaders can’t trust their data, they delay making critical decisions. Real-time market changes, competitor moves, or emerging consumer trends may go unaddressed.
How Data Parsing Creates Actionable, Structured Data
Data parsing addresses these challenges by transforming raw data into usable formats like JSON, XML, or CSV. The data is also cleaned to remove duplicates, errors, and inaccuracies and to fill in missing values or essential IDs and tags for organization.
By extracting relevant information and organizing it into structured datasets, companies can generate actionable insights.
The Competitive Advantages of Structured Data
Faster Decision-Making
Parsed data enables real-time analysis, allowing businesses to respond quickly to market changes.
Personalization at Scale
Structured customer data enhances personalized marketing efforts by providing clear insights into customer behavior, preferences, and purchase history. Retailers can offer tailored product recommendations, financial institutions can customize loan offers, and media platforms can suggest relevant content to achieve higher engagement and customer satisfaction.
Improved Analytics and Forecasting
Clean, structured data ensures that predictive models and machine learning algorithms work with accurate inputs. Businesses gain better demand forecasts, pricing predictions, and risk assessments.
Operational Efficiency
With structured data flowing seamlessly into analytics tools and business intelligence platforms, teams can focus on high-value tasks like strategy development rather than data cleaning.
Enhanced Data Integration
Structured data is easier to merge and analyze across different systems. Businesses can combine data from CRMs, e-commerce platforms, and third-party APIs for a comprehensive view of their operations, leading to better cross-functional collaboration and faster insights.
Compliance and Risk Management
Regulatory compliance becomes easier when businesses work with clean, structured data. Accurate reporting, transparent data audits, and faster anomaly detection reduce the risk of non-compliance and ensure data governance standards are met.
Want to simplify your data extraction and data parsing process? Try Nimble’s Web API for free.
Key Use Cases of Data Parsing Across Industries
Data parsing plays a crucial role in making data accessible and functional across industries. Here are a few examples of how data parsing fuels better strategies in retail & e-commerce, finance, real estate, and the AI industries.
Retail & Ecommerce
Retailers and e-commerce companies rely on data parsing to make informed decisions regarding pricing, inventory, and customer engagement. Here’s how it helps:
- Price Monitoring and Dynamic Pricing: By parsing competitor pricing data, retailers can adjust their prices in real time to stay competitive. This is especially valuable in marketplaces where pricing fluctuates based on demand.
- Inventory Management: Parsed data helps businesses track stock levels, anticipate restocking needs, and optimize supply chain logistics.
- Customer Sentiment Analysis: Parsing unstructured customer reviews and social media mentions allows businesses to gauge public sentiment and refine their marketing strategies.
Competitor Intelligence: By extracting data from multiple sources like competing brand websites or large e-commerce sites like Amazon, businesses can analyze competitors’ product offerings, promotions, and pricing strategies to maintain an edge.
See how Nimble’s Data Parsing Enhances E-commerce Strategies with Nimble’s E-commerce Scraping API.
Finance
Financial institutions leverage data parsing for fraud detection, market analysis, and regulatory compliance. Structured data ensures accuracy and efficiency in financial decision-making.
- Fraud Detection: Parsing transaction data helps identify suspicious patterns that could indicate fraudulent activities.
- Market Analysis: Investors use data parsing to monitor stock prices, earnings reports, and financial news articles to make informed investment decisions.
Regulatory Compliance: Financial institutions must comply with strict regulations. Parsing data from reports and transactions ensures accurate auditing and reporting. - Customer Insights: Financial institutions analyze spending behaviors through parsed transaction data to offer personalized services and promotions.
Get Instant Access to Market Data–Learn More About Nimble for Finance.
Real Estate
In the real estate sector, businesses collect vast amounts of unstructured data from property listings, pricing databases, and market reports. Data parsing helps turn this information into actionable insights.
- Property Listings Aggregation: Aggregating listings from multiple sources allows real estate platforms to provide comprehensive property databases.
- Market Trend Analysis: Parsing historical sales data and property valuations helps investors track trends and make informed investment decisions.
- Competitor Monitoring: Real estate agencies can analyze competitors’ pricing strategies and listings to refine their offerings.
- Lead Generation: Extracting contact information from listing platforms helps real estate businesses identify potential buyers and sellers.
AI and Machine Learning
Data parsing is a foundational step in training machine learning models, as it ensures clean, structured input data.
- Data Preprocessing: Parsed data is used to train AI models, ensuring consistency and accuracy in datasets.
- NLP Applications: Businesses use text parsing for applications like chatbots, sentiment analysis, and content moderation.
- Computer Vision: Parsing metadata from images and videos extracted via bulk SERP processing helps improve AI-driven image recognition models.
- Predictive Analytics: Structured data enhances forecasting models, making them more accurate in various domains, from retail demand forecasting to financial predictions.
Tools and Best Practices for Effective Data Parsing
Implementing data parsing effectively requires the right tools and strategies. Businesses can either build custom parsing solutions, leverage pre-built tools for efficiency, or do a mix of both.
Common Data Parsing Tools
Here’s a breakdown of the different types of data parsing solutions that are available.
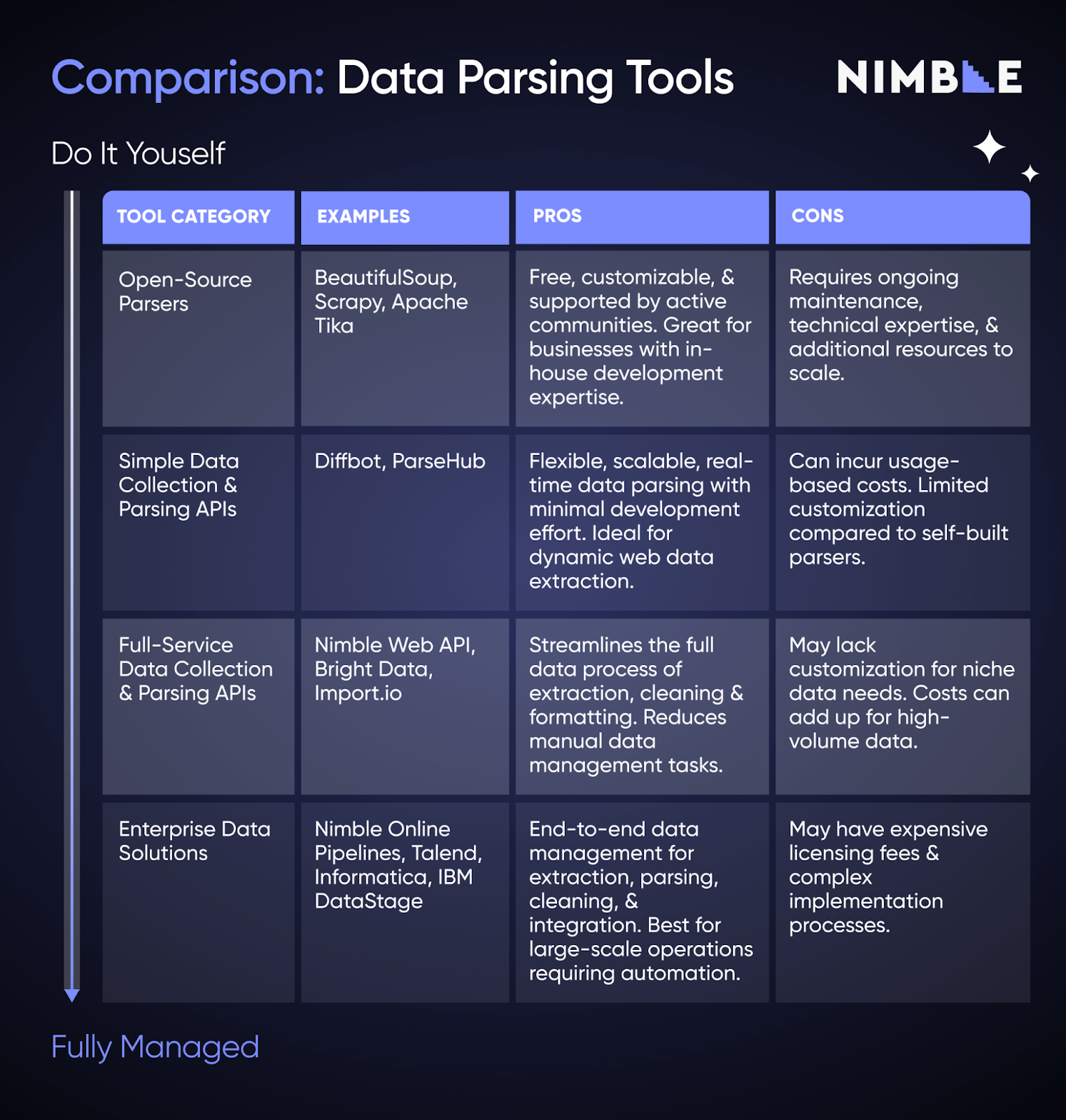
To learn more about how to build data parsing tools yourself, read:
What to Look for in a Parsing Solution
When selecting a data parsing tool, businesses should consider:
- Scalability: The ability to handle large volumes of data without performance bottlenecks.
- Automation Features: AI-powered parsing tools that reduce manual intervention.
- Data Quality Management: Built-in validation and error detection mechanisms.
- Real-Time Capabilities: Support for instant data extraction and updates.
Nimble’s Web API is a powerful solution that automates large-scale data parsing for businesses needing structured, real-time data extraction, while Nimble’s Online Pipelines manages your entire data collection, parsing, and analysis system from end to end.
Find out what Nimble solution works best for you—Book a Demo Now.
Tips for Integrating Data Parsing Into Your Business Workflow
To maximize the benefits of data parsing, businesses should:
- Start Small: Implement parsing on a key data source before scaling.
- Automate Gradually: Use AI-based solutions to expand parsing capabilities efficiently.
- Monitor Data Quality: Continuously validate parsed data to ensure accuracy.
- Ensure Flexibility: Choose APIs that support multiple data formats for seamless integration.
How Automation Enhances Data Parsing
Automating data parsing eliminates inefficiencies and enables businesses to scale data processing without manual intervention, allowing for a boost in speed, cost savings, and accuracy.
AI-Driven Parsing for Large Datasets
AI-powered parsing tools like Nimble’s parsing skills can recognize patterns, extract relevant data, and clean inconsistencies. This ensures that businesses can process millions of data points in real time without human intervention.
API-Based Data Extraction
APIs like Nimble’s Web API make it easy to extract data from various sources and integrate structured data into existing business workflows.
Conclusion
Data parsing is the key to converting raw, unstructured data into actionable insights. Businesses that leverage structured, real-time data can enhance decision-making, improve operational efficiency, and stay ahead of competitors.
Nimble’s Web API provides an all-in-one solution for automated, scalable data parsing, making it easier for businesses to extract high-quality data in real time.
Book a demo with Nimble to experience the power of automated data parsing in action.
FAQ
Answers to frequently asked questions